Early Phase Performance Driven Design Assistance Using Generative Models
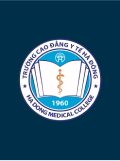
Xem mô tả
0
Xem & Tải
0
Tóm tắt
Mô tả
Form-finding in the current performance-driven design methodology of architectural design is
typically formulated as a design optimization problem. Although effective in engineering or late-stage
design problems, optimization is not suitable for the exploratory design phase due to the
time intensity and cognitive load associated with the processes involved in the formulation and
solution of optimization problems. The iterative, diverging nature of early-phase design is
incompatible with the i) cognitive load of parametric modeling and its limited affordances for
conceptual changes, ii) time and resource intensity of simulations, iii) interpretability of
optimization results.
This thesis suggests a framework for generating optimal performance geometries within an
intuitive and interactive modeling environment in real-time. The framework includes the
preparation of a synthetic dataset, modeling its probability distribution using generative models,
and sampling the learned distribution under given constraints. The several components are
elaborated through a case study of building form optimization for passive solar gain in Boston,
MA, for a wide range of plot shapes and surroundings. Apart from the overall framework, this
thesis contributes a series of methods that enable its implementation. A geometric system of
orientable cuboids is introduced as a generalizable, granular modeling vocabulary. A method for
efficient boundary condition sampling is suggested for the dataset preparation. A Variational
Autoencoder (VAE) is extended for performance-aware geometry generation using performance-related
loss functions. A series of techniques inspired by the data-imputation literature is
introduced to generate optimal geometries under constraints. Last, a prototype is presented that
demonstrates the abilities of a system based on the suggested framework.
Năm xuất bản
Tác giả
Ampanavos, Spyridon